There are an estimated 15 million deaths caused by incorrect or delayed diagnosis every year, according to the World Health Organization. AI could give doctors insights into increasing patient information, such as medical history and laboratory test results.
Artificial Intelligence (AI) has been making significant strides in healthcare, with multimodal AI leading the charge. Multimodal AI refers to the use of AI that integrates data from multiple sources or modalities, such as medical imaging, electronic health records, wearable biosensors, and more, to provide a comprehensive understanding of human health and disease. This approach enables more holistic patient assessments, drawing from diverse data sources for accurate diagnoses and treatment recommendations.
Multimodal AI in Healthcare
In Healthcare, multimodal AI can integrate data from multiple sources to create a more accurate diagnosis. For example, a multimodal AI system can integrate data from various image types (MRI, CT, PET) to improve the accuracy of diagnosis and proposed treatment.
For instance, the Holistic AI in Medicine (HAIM) framework developed by MIT researchers leverages multiple data sources to build forecast models more easily in healthcare settings. The HAIM framework uses generalizable data pre-processing and machine learning modeling stages that can be readily adapted for research and deployment in healthcare environments.
The Role of Multimodal AI in Holistic Patient Assessment
The use of multimodal AI in healthcare allows for a more comprehensive and holistic patient assessment. By integrating data from various sources, multimodal AI can provide a more complete picture of a patient’s health status. This can lead to more accurate diagnoses, more effective treatment plans, and ultimately, better patient outcomes.
Multimodal AI can assist in analyzing complex associations and relationships between various biological processes, health indicators, risk factors, and health outcomes, and developing exploratory and explanatory models in parallel. This can lead to more personalized and precise care, improving disease detection and prediction, and streamlining administrative processes.
The Advantages of HAIM framework
The HAIM framework addresses several bottleneck challenges in AI/ML pipeline for healthcare in a more unified and robust way than previous implementations. It can work with tabular and non-tabular data of unknown sparsity from multiple standardized and unstandardized heterogeneous data formats.
The use of multimodal AI in healthcare allows for a more comprehensive and holistic patient assessment. By integrating data from various sources, multimodal AI can provide a more complete picture of a patient’s health status. This can lead to more accurate diagnoses, more effective treatment plans, streamlining administrative processes and ultimately, better patient outcomes.
The Holistic AI in Medicine (HAIM) framework developed by MIT researchers is a promising tool for the future of AI physicians. It leverages multimodal inputs, including tabular data, time-series data, text, and images, to generate and test AI systems in healthcare.
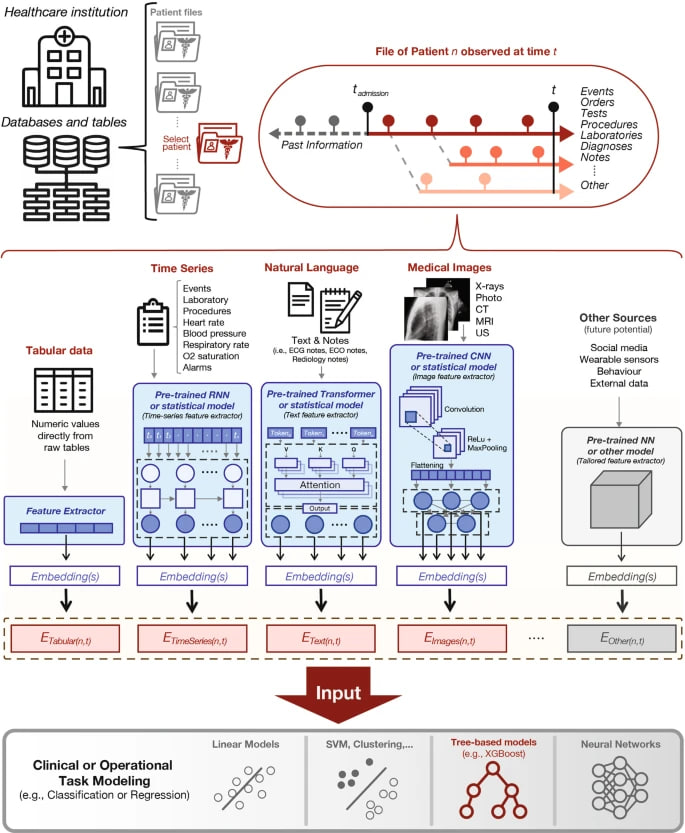
The self-learned AI Physicians
The HAIM framework addresses several bottleneck challenges in AI/ML pipeline for healthcare in a more unified and robust way than previous implementations. It can work with tabular and non-tabular data of unknown sparsity from multiple standardized and unstandardized heterogeneous data formats.
AI Physicians could be based at HAIM ideas to conduct holistic patient assessments. For instance, an AI physician could integrate data from various sources such as medical images, patient medical history, real-time health data from wearable devices, and even genomic data. This would allow it to have a comprehensive understanding of the patient’s health status, similar to how a human physician would assess a patient.
This could potentially lead to the development of AI physicians that can provide more accurate diagnoses and predictions, improving patient outcomes and quality of care. However, further research and development are needed to address the challenges associated with implementing this framework in real-world healthcare settings.
Multimodal AI for ambulance paramedics
Real-time decision support: Ambulance paramedics could use AI to integrate and analyze various types of data, including patient medical history, vital signs, images from portable medical devices, and even recording patient symptoms, in real-time. This could help paramedics make more accurate assessments and decisions in emergency situations. AI could provide additional insights based on its analysis of multimodal data in complex or ambiguous cases.
Enhancing triage and diagnosis: AI algorithms can be used to quickly assess patients and prioritize their treatment based on the severity of their condition, potentially improving patient outcomes
Mitigating human error and reducing workload: AI can help reduce the risk of human error by automating certain tasks and providing decision support, allowing paramedics to focus on critical aspects of patient care.
Quality improvement initiatives: AI can analyze large healthcare datasets to identify patterns, trends, and areas for improvement, empowering ambulance services to enhance their protocols, optimize workflows, and continuously improve the quality of care delivered
AI-enabled dispatch systems: AI can be used to improve dispatch systems by leveraging real-time data analysis and predictive models to determine the optimal response strategy, ensuring that the nearest and most appropriate ambulance is dispatched promptly.
The use of multimodal AI in healthcare is revolutionizing the way patient assessments are conducted. By integrating data from multiple sources, multimodal AI provides a more holistic and comprehensive understanding of a patient’s health, leading to more accurate diagnoses and more effective treatment plans.